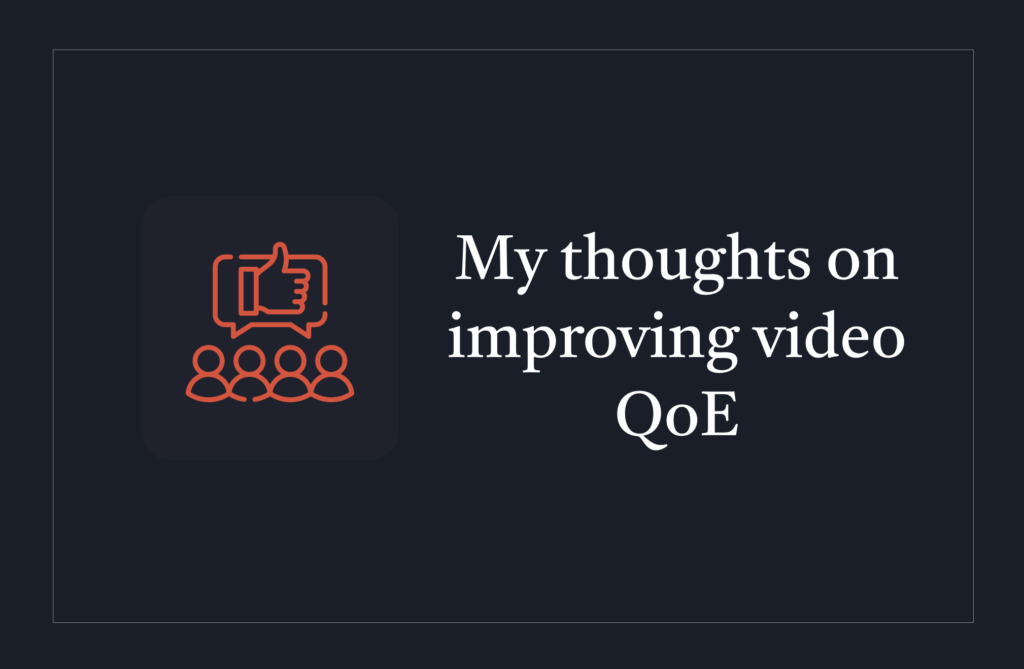
Some years ago, five Brazilian researchers published a paper called “Video Quality Metric for Streaming Service Using DASH Standard”1. The paper aimed to propose a new, non-reference video quality metric, which they coined the Video streaming Quality Metric for DASH, or VsQMDASH, which would assess the impact of the DASH standard on end viewers’ quality of experience (QoE). In order to reach a conclusion, the researchers took the following parameters into account:
The number, length, and temporal location of each pause during a given video sequence,
The number and temporal location of video quality switching events, switching types that consider temporal and spatial resolutions; and,
Initial buffering delay.
Quality of service vs. quality of experience
Interestingly, the researchers commented how over the past two decades, the quality of multimedia services has been rated solely against the idea of quality of service (QoS) —not on quality of experience. However, the correlation between QoS and user satisfaction was seldom taken into account. When the quality of experience was drawn into the equation, the correlation seemed to improve2. In a paper published by A. Raake and S. Egger, QoE is defined as “the degree of delight or annoyance of a person whose experience involves an application, service, or system. It results from the person’s evaluation of fulfilling their expectations and needs with regard to the utility and/or enjoyment in the light of the person’s context, personality, and current state”3.
In order, therefore, to determine this new metric and its ability to help enhance QoE, and evaluate its performance, the researchers conducted a study. For the present research project, potential QoE impact factors were divided into three categories: system, context, and human. System-related impact factors refer to properties and characteristics that determine the technically produced quality of an application or service; context-related impact factors refer to physical, temporal, social, and economic contexts, and human-related impact factors refer to viewers’ individual characteristics, or cognitive processes, such as memory, categorization and attention.
The methodology
Each of the above impact factor categories was tested. Switching events and temporal interruptions or pauses were classed as system-related; all participants were placed in the same physical environment thus all were influenced by the same context factors. Context-related impact factors were also tested by showing the videos on different devices, namely a PC and a tablet. Finally, any pauses (and their temporal location) could also interrupt viewers’ cognitive mechanisms, and as such, human impact factors were also taken into account.
Subjective tests were conducted to establish the VsQMDASH model’s effectiveness. In an 18-week experiment in an unchanged test room, 119 participants evaluated video quality on a 21.5-inch LCD monitor. Participants individually rated videos lasting 60 or 90 seconds, with initial delays ranging from 2 to 22 seconds. Participants noted their responses using a scale. Statistical analysis showed no outliers. The subjective results indicated that initial buffering delays significantly affected viewers’ QoE. In fact, the impact of impairments in the initial video segment was found to be more negative than in the intermediate and final segments. The negative impact of initial pauses could be influenced by participants’ experiences streaming video services, potentially resulting in biased, prior expectations.
A high-performing, promising metric
In assessing the model’s performance, they found that it performed very highly, with negligible impact on viewers’ devices regarding processing and energy consumption. They also found that its low level of complexity means it can be used to assess viewers’ QoE on hand-held mobile devices, a hugely important consideration given that almost 60% of the total mobile data traffic is now generated by mobile devices4.
Furthermore, they deemed the VsQMDASH able to work with current video content providers that consider several video resolutions and improve the performance of DASH control algorithms.
Most importantly, however, they found that the metric takes into account changes in video resolution and quality that occur throughout the history of each viewer session, a key component that impacts overall QoE. Measuring the frequency of these quality changes is a vital step in determining the quality of a streaming provider’s service, and thus their ability to acquire and retain their audience.
Overall, the findings positioned VsQMDASH as a very promising metric for assessing video quality and elevating the video streaming experience, as well as offering a glimpse into the future of adaptive and immersive multimedia services.
The world of streaming has evolved exponentially over the past twenty years, both in terms of services and the tools and methodologies implemented to measure the quality of these services, such as VsQMDASH. Looking ahead to the future, at System73, we will continue to align ourselves with this pace of evolution and analysis, working on optimizing our services and analytics solutions and helping our clients enhance their content delivery networks, with a view to offering the best QoE to end viewers.
- D. Zegarra Rodríguez, R. Lopes Rosa, E. Costa Alfaia, J. Issy Abrahão, and G. Bressan, “Video Quality Metric for Streaming Service Using DASH Standard”. Published in IEEE Transactions On Broadcasting, Vol. 62, No. 3, September 2016. Pp. 628-639 ↩︎
- Ibidem, p.630 ↩︎
- A. Raake and S. Egger, “Quality and quality of experience,” in Quality of Experience: Advanced Concepts, Applications and Methods, S. Möeller and A. Raake, Eds. Berlin, Germany: Springer, 2014, ch. 2, pp. 11–33. ↩︎
- https://www.statista.com/statistics/277125/share-of-website-traffic-coming-from-mobile-devices/ ↩︎